

TACAS, April 2016, Eindhoven, Netherlands
#MAX 4 LIVE OTOMATA VERIFICATION#
Invariant Synthesis for Incomplete Verification Engines.Invited talk, June 2018, Saarland University, Germany Machine Learning Meets Formal Methods.Invited tutorial at MOVEP '18 Summer School, July 2018, ENS Paris-Saclay, France Invited talk, Highlights of Logic, Games and Automata, September 2018, Berlin, Germany Horn-ICE Learning for Synthesizing Invariants and Contracts.Invited talk, Complexity, Algorithms, Automata and Logic Meet, January 2019, Chennai Mathematical Institute, India Invited talk, March 2019, University of Bochum, Germany Formal Verification Meets Machine Learning.Invited tutorial at the ForMaL Spring School onįormal Methods and Machine Learning, June 2019, ENS Paris-Saclay, France

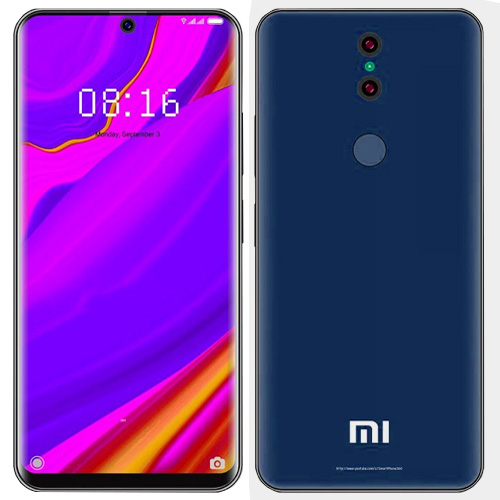
Deductive Verification, the Inductive Way.Sorcar: Property-Driven Algorithms for Learning Conjunctive Invariants.Invited talk, October 2019, University of California, Los Angeles, USA Combining Deductive and Inductive Reasoning in Formal Methods.Invited talk, January 2020, University of Liverpool, UK Learning Correctness Proofs of Software.Invited talk, November 2020, University of Oldenburg, Germany Logic and Learning: Formal Guarantees for Trustworthy Intelligent Systems.Invited talk, June 2021, RWTH Aachen University, Germany
#MAX 4 LIVE OTOMATA SOFTWARE#
#MAX 4 LIVE OTOMATA CODE#
Learning-based synthesis: automatically generating reactive systems and program code from logical specifications and examples.Intelligent formal methods: tools that learn correctness and termination proofs for a wide range of systems, including hardware, software, and cyber-physical systems.Explanability of artificial intelligence: generating provably correct explanations for the decision making of intelligent systems.Verification of learning systems: automatically proving correctness properties (e.g., robustness) of learning systems.My group is working on a number of topics, including: The goal of my group is to build automated tools for the design, the construction, and the analysis of intelligent systems. I am especially interested in combining inductive techniques from the area of machine learning and symbolic techniques from the area of logic. My research interests broadly lie in the intersection of machine learning and formal methods. Moreover, I am principal investigator in several research projects. I am a research group leader at the Max Planck Institute for Software Systems in Kaiserslautern and teach at the University of Kaiserslautern (see below a list of course I offer). I have moved to the Carl von Ossietzky Universität Oldenburg, where I am now professor for Safety and Explainability of Learning Systems.
